Gen Z Data Scientist Reveals AI Jobs Demand More Than Just Coding — and Teamwork Is Essential
Discover why AI jobs involve more than coding as Pranjali Ajay Parse discusses the necessity for teamwork, interdisciplinary skills, and ethical considerations in AI careers.
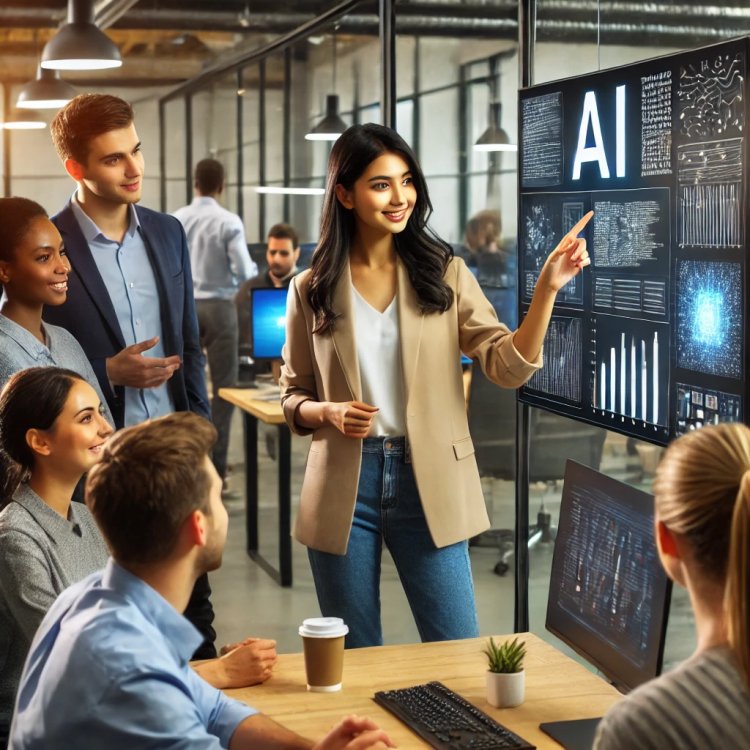
There’s More to AI Jobs Than Just Coding
Pranjali Ajay Parse, a 25-year-old data scientist at Autodesk, has gained valuable insights into what it's really like to work in AI. Over the past year, she’s been developing an AI tool to help employees better understand their work habits, such as meeting patterns and daily routines.
With a master's degree in computer science and her experience at Autodesk, Parse has realized that working in AI is not what many might expect. It's a field that goes beyond coding and demands interdisciplinary skills and collaboration.
AI Is Not Just About Coding
Parse emphasizes that proficiency in Python alone won’t land you a job in AI. While you don’t necessarily need a degree in AI, understanding case study analysis, SQL querying, and coding is crucial. She suggests that aspiring AI professionals can build these skills through boot camps or personal projects.
“AI is inherently interdisciplinary,” says Parse. “It draws from various domains, including mathematics, computer science, statistics, and domain-specific knowledge.”
In her role, about 70% of Parse's work involves data science, focusing on reviewing and analyzing datasets. The remaining time is spent on software engineering, pipeline building, data engineering, architectural design, and applying mathematical concepts. Staying current with technological advancements is also essential in this rapidly evolving field.
Collaboration Is Key in AI Roles
Unlike the stereotype of the solitary software engineer, AI roles are often highly collaborative. Parse points out that AI projects are rarely completed solo because they require input from multiple teams and stakeholders.
For instance, in one AI recommendation system project, Parse collaborated with seven or eight different teams. The process started with data collection and preparation by a data analysis team, followed by statistical modeling by data scientists. The machine learning team then refined the model, UX/UI experts designed the user interface, and software engineers built the front end. Finally, the marketing team developed the product’s launch strategy.
“An end-to-end AI project requires a lot of communication and collaboration,” Parse explains.
Ethics Is a Major Consideration
When AI projects involve sensitive data, privacy teams play a critical role. Parse describes the extensive privacy protocols in place, including obtaining consent for data usage, pseudonymizing identities, and ensuring that models do not perpetuate biases or create unfair outcomes.
These efforts must align with legal and regulatory standards while also considering the long-term implications and potential ethical challenges of AI projects.
While privacy may seem like an obvious concern, Parse warns that it’s easy to focus solely on model performance and lose sight of the broader ethical implications. Given the collaborative nature of AI work, it’s essential for both companies and employees to remain vigilant about privacy and ethics throughout the project lifecycle.
Parse believes that while companies should provide training on privacy and ethical guidelines, it’s equally important for employees to consider the impact of their work from a third-person perspective.
- Read the full article on our site: Programmers Press
- Learn more about AI services: Explore AI solutions