The Data That Powers A.I. Is Disappearing Fast
The availability of large datasets, crucial for AI development, is rapidly declining due to stringent data privacy regulations, proprietary data hoarding by tech giants, and legal challenges surrounding copyrighted materials. This scarcity poses significant challenges for future AI advancements.
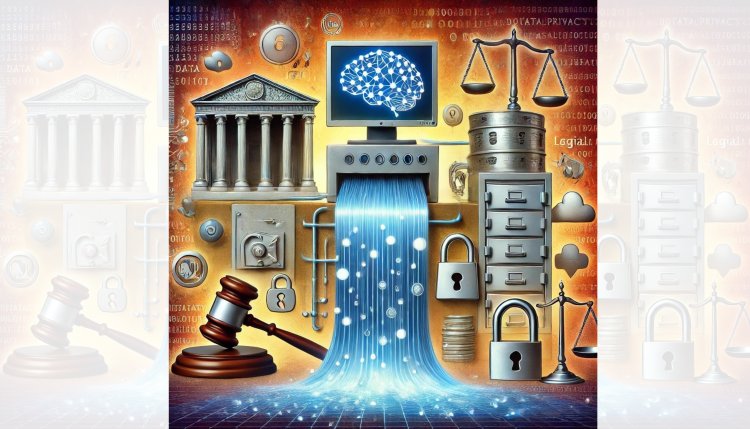
In the rapidly evolving field of artificial intelligence (AI), access to large and diverse datasets has been a cornerstone for developing advanced models. However, a troubling trend has emerged: the data that powers AI is disappearing at an alarming rate. This scarcity poses significant challenges for future advancements and innovation in AI.
The Impact of Data Privacy Regulations
One of the primary drivers of this data scarcity is the increasing stringency of data privacy regulations globally. Laws such as the General Data Protection Regulation (GDPR) in Europe and the California Consumer Privacy Act (CCPA) in the United States have set stringent standards for data collection, usage, and sharing. These regulations aim to protect individuals' privacy and personal data but inadvertently limit the availability of datasets for AI research and development (Harvard Gazette).
Proprietary Data Hoarding by Tech Giants
Another contributing factor is the growing trend among tech giants to hoard data. Companies like Google, Facebook, and Amazon possess vast amounts of user data but are often reluctant to share it due to competitive advantages and proprietary interests. This data hoarding exacerbates the scarcity problem, making it difficult for smaller companies and independent researchers to access the data necessary for developing innovative AI solutions (Our World in Data).
Legal and Ethical Challenges
The legal landscape surrounding the use of copyrighted materials in AI training also presents significant hurdles. Numerous disputes and litigations have arisen over the unauthorized use of books, images, and other creative works for training AI models. These legal challenges create a complex and risky environment for obtaining and utilizing large datasets, further contributing to the data scarcity (Harvard Gazette).
Consequences for AI Development
The implications of this data scarcity are profound. AI models rely heavily on large, high-quality datasets to learn and improve their performance. Without access to such data, the accuracy and reliability of AI systems can be severely compromised. This limitation could slow down progress in various fields where AI has shown promise, such as healthcare, autonomous driving, and natural language processing (Our World in Data).
Potential Solutions
Addressing the issue of data scarcity in AI will require a multifaceted approach. Some potential solutions include:
-
Enhanced Data Anonymization: Developing advanced anonymization techniques can help protect individual privacy while enabling access to valuable data for AI research.
-
Collaborative Data Sharing: Encouraging collaborations between tech companies, academic institutions, and governments can foster a more open data ecosystem. Sharing data under strict ethical guidelines can mitigate competitive concerns while promoting innovation.
-
Synthetic Data Generation: Investing in technologies that generate synthetic data, which mimics real-world data, can provide an alternative source for training AI models. This approach can alleviate some of the pressures from data scarcity while ensuring privacy and compliance with regulations.
-
Regulatory Adjustments: Policymakers can consider revising existing data protection regulations to strike a better balance between privacy concerns and the need for data in AI development. Implementing frameworks that facilitate safe and ethical data sharing can help bridge the gap.
Conclusion
The disappearing data that powers AI is a pressing issue that threatens to hinder the advancement of artificial intelligence. By addressing the challenges of data privacy, proprietary data hoarding, and legal complexities, the AI community can work towards solutions that ensure a steady flow of data for innovation. A collaborative and ethical approach to data management will be essential in sustaining the growth and potential of AI technologies in the coming years.